User intent in retail refers to the underlying motivation or purpose behind a customer’s actions or behavior when interacting with a retail brand, whether online or in-store.
It’s about understanding why a customer is searching for a product, browsing a category, or engaging with your brand.
Understanding and predicting user intent is crucial for enhancing customer experience and driving sales in retail for several reasons:
- Personalized Shopping Experience:
- When detecting user intent, retailers can provide personalized recommendations, relevant content, and targeted offers that resonate with the customer’s current needs and desires. This personalization makes the shopping experience more engaging and satisfying, increasing the likelihood of conversion.
- Improved Customer Satisfaction:
- When retailers understand what a customer is looking for, they can streamline the shopping process, making it easier and faster for the customer to find what they need. This reduces frustration and improves overall satisfaction, leading to repeat business and customer loyalty.
- Higher Conversion Rates:
- Predicting transactional intent allows retailers to strategically place calls-to-action, promotional offers, and incentives at the right moment in the customer journey, significantly boosting the chances of a sale.
- Efficient Marketing Spend:
- Segmenting and targeting customers based on their intent, retailers can optimize their marketing efforts, ensuring that resources are spent on the right audience at the right time. This leads to better ROI on marketing campaigns and more effective use of budgets.
- Competitive Advantage:
- Retailers who excel at understanding and acting on user intent can differentiate themselves in a crowded market. They can anticipate customer needs, offer superior service, and stay ahead of competitors by delivering exactly what customers are looking for.
- Enhanced Data-Driven Decisions:
- Predicting user intent helps retailers make informed decisions based on data-driven insights. This can influence everything from inventory management to product development and pricing strategies, ensuring that the business is aligned with customer demand.
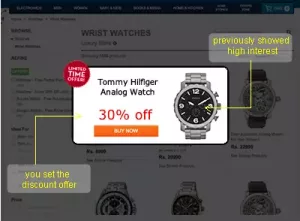
Understanding User Intent in Retail
In retail, user intent typically falls into three broad categories:
- Navigational Intent: The customer is looking to find a specific product, brand, or category. For example, a shopper searching for “Nike running shoes” is likely intending to find a specific product or brand within that category.
- Informational Intent: The customer is seeking information or answers to questions. This could involve looking for product reviews, comparison articles, or guides. For example, a user searching for “best running shoes for flat feet” is likely gathering information before making a purchasing decision.
- Transactional Intent: The customer has the intention to make a purchase. This intent is the most direct and indicates that the customer is close to completing a transaction. For example, a search for “buy Nike running shoes size 10” shows clear intent to make a purchase.
Examples in Retail Context:
1. Navigational Intent:
- Scenario: A customer searches for “Nike Air Max size 10.”
- Understanding Intent: The user knows exactly what they want and is likely looking for that specific product.
- Improving Outcomes: By understanding this clear intent, the retailer can ensure that the search results show the exact product, available sizes, and purchasing options prominently. Offering quick access to product availability, store locations, and a seamless checkout process can increase the likelihood of a purchase.
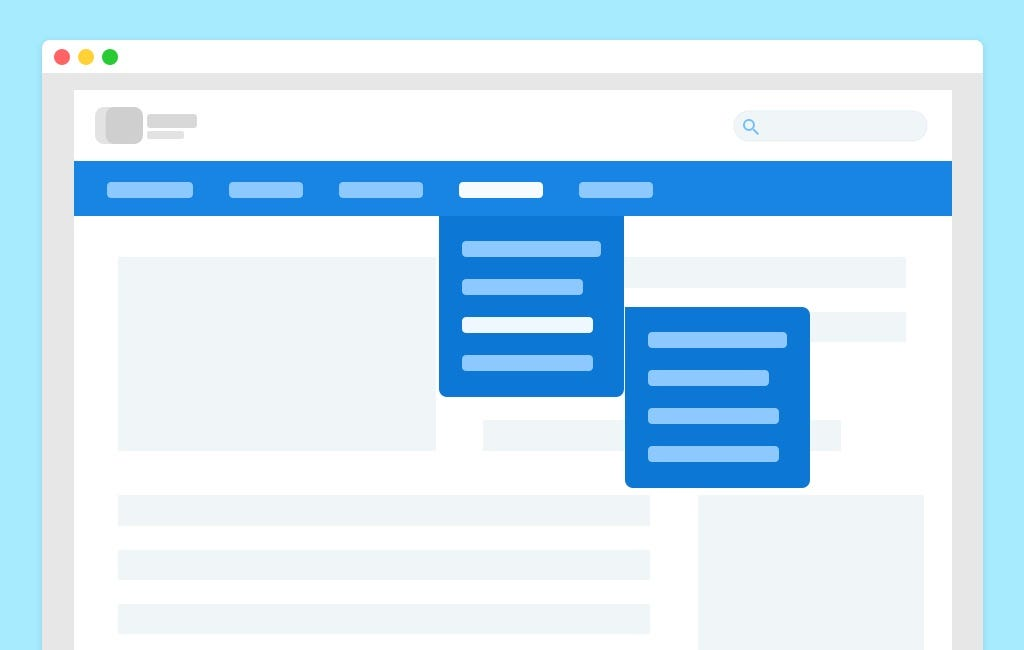
2. Informational Intent:
- Scenario: A customer searches for “best running shoes for flat feet.”
- Understanding Intent: The user is in the research phase, gathering information before making a purchase.
- Improving Outcomes: The retailer can improve outcomes by providing relevant content, such as a blog post or guide on choosing the best running shoes for flat feet. Additionally, product recommendations based on the information provided can guide the customer towards making a well-informed purchase.
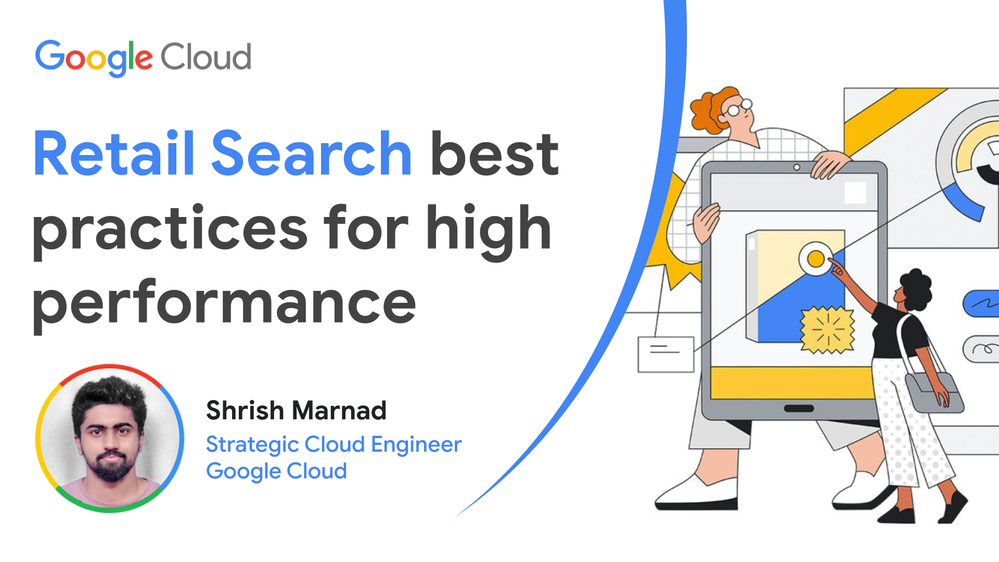
3. Transactional Intent:
- Scenario: A customer searches for “buy Nike running shoes size 10.”
- Understanding Intent: The user is ready to make a purchase and is looking for the best place to buy the product.
- Improving Outcomes: By recognizing this strong transactional intent, the retailer can present direct purchase options, clear pricing, and promotions such as discounts or free shipping. Ensuring an easy checkout process and providing options like “buy now” buttons or a quick checkout link can seal the deal.
4. Cross-Sell/Up-Sell Intent:
- Scenario: A customer adds a pair of running shoes to their cart.
- Understanding Intent: The user is in the purchasing process and may be open to related products.
- Improving Outcomes: The retailer can suggest complementary products like running socks, insoles, or athletic apparel during the checkout process. By understanding the customer’s intent to purchase, targeted cross-sell or up-sell offers can increase the average order value.
5. In-Store Visit Intent:
- Scenario: A customer searches for “Nike store near me.”
- Understanding Intent: The user is looking to visit a physical store, possibly to try on or purchase a product.
- Improving Outcomes: The retailer can provide detailed store information, including hours, directions, and in-store promotions. Additionally, offering options like reserving a product online for in-store pickup can enhance the customer’s shopping experience and ensure the visit results in a purchase.
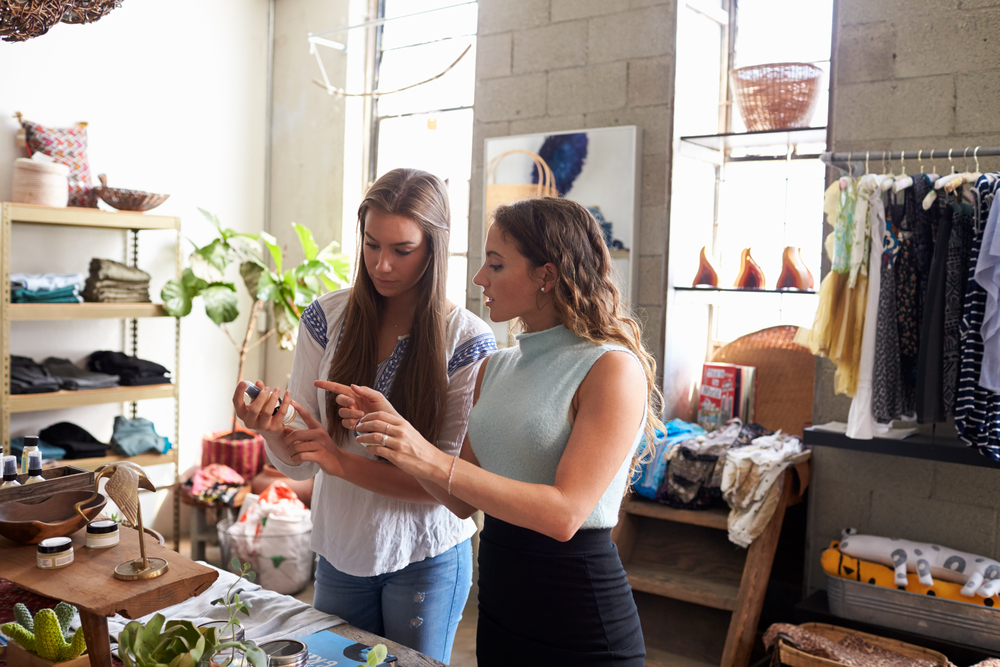
6. Brand Discovery Intent:
- Scenario: A customer searches for “eco-friendly fashion brands.”
- Understanding Intent: The user is exploring brands that align with specific values, such as sustainability.
- Improving Outcomes: The retailer can highlight their eco-friendly product lines, provide information about their sustainability practices, and offer incentives like discounts on the first purchase. This approach can help capture the customer’s interest and convert it into brand loyalty.
Techniques for Detecting User Intent
There are multiple techniques that marketers use to detect user intent. The most common ones are:
Analyzing Search Queries
How Search Behavior Reveals Intent (Keywords, Phrases):
- Keywords and Phrases: When customers use specific keywords or phrases in their search queries, they provide direct clues about their intent. For example, someone searching for “cheap running shoes” is likely price-sensitive and looking for budget options, whereas a search for “premium running shoes” indicates a willingness to spend more for quality.
- Long-Tail Keywords: These are more specific phrases, often with higher purchase intent. A search like “best running shoes for marathon training” shows a clear intent to find a product that meets a particular need, suggesting that the user is further along in the decision-making process.
- Contextual Understanding: Beyond just the keywords, understanding the context of the search can provide deeper insights. For example, a search for “buy Nike shoes” near a major shopping holiday might indicate urgency and a readiness to purchase.
Analyzing the keywords and phrases customers use, retailers can tailor their search results, product recommendations, and even promotional offers to better match the user’s intent, thereby increasing the likelihood of conversion.
Behavioral Analysis
Tracking User Behavior on the Website (Clicks, Time Spent on Pages, Cart Activity):
- Click Patterns: Analyzing what links or products users click on provides insights into their interests and preferences. If a user clicks on multiple items within a specific category, it suggests a strong interest in that category.
- Time Spent on Pages: The amount of time a user spends on a particular page can indicate the level of interest or engagement. For instance, spending several minutes on a product detail page might suggest a higher intent to purchase that product.
- Cart Activity: Monitoring actions such as adding items to the cart, removing them, or abandoning the cart altogether can reveal intent. A user who frequently abandons their cart might be price-sensitive, indicating that targeted discounts or free shipping offers could convert them into a buyer.
- Bounce Rate and Exit Pages: High bounce rates or frequent exits from certain pages might indicate that the content or product doesn’t match user intent, providing an opportunity to optimize those pages or offerings.
Tracking and analyzing these behaviors, retailers can adjust their website layout, content, and marketing strategies to better align with what customers are looking for, thus enhancing the shopping experience and driving sales. Note that for tracking you need a data platform.
Use of AI and Machine Learning
Leveraging AI to Detect Patterns and Infer Intent from Complex Data:
- Pattern Recognition: AI can analyze vast amounts of data to detect patterns that are not immediately obvious. For example, machine learning algorithms can identify correlations between search queries, browsing behavior, and purchase history to predict what a customer might be interested in next.
- Personalization Engines: AI-driven personalization tools can deliver tailored content and product recommendations based on real-time analysis of user behavior. For example, if a user frequently searches for eco-friendly products, the system can prioritize displaying green or sustainable products to that user.
- Predictive Analytics: Machine learning models can be trained to predict future behaviors based on past actions. For example, if a customer typically makes a purchase after visiting a product page three times, AI can recognize this pattern and trigger targeted ads or emails after the second visit to encourage conversion.
- Natural Language Processing (NLP): AI can analyze the language used in search queries and customer reviews to detect sentiment and intent. NLP allows the system to understand not just what the user is searching for, but also how they feel about it, which can be critical for personalizing the customer experience.
AI and Machine learning helps with this a lot. At Vibetrace we’ve been using ML for more than 10 years to build our recommender engine.
Using those retailers can move beyond basic data analysis to more sophisticated, real-time predictions of user intent, enabling them to offer highly personalized and relevant shopping experiences.
Utilizing Historical Data
Analyzing Past Interactions to Predict Current Intent:
- Purchase History: Reviewing what a customer has bought in the past can provide clues to their future needs. For example, a customer who frequently buys running gear might be interested in the latest athletic wear, allowing for timely, targeted marketing.
- Browsing Patterns: Analyzing the pages or products a customer has viewed over time can help predict what they might be looking for next. If a user consistently browses a particular category without purchasing, it may suggest interest but hesitation, possibly due to price or uncertainty, which can be addressed with targeted offers or additional information.
- Search History: A customer’s search history provides a direct insight into their evolving needs and interests. By analyzing past searches, retailers can predict what the user might search for next and preemptively offer related products or content.
- Customer Segmentation: Historical data can be used to segment customers into different groups based on their behavior, preferences, and purchasing patterns. This segmentation allows for more precise targeting and personalized marketing strategies that align with the predicted intent of each segment.
Utilizing historical data allows retailers to understand the long-term behavior and preferences of their customers, enabling them to anticipate needs and provide personalized experiences that resonate with each individual user. This proactive approach not only enhances customer satisfaction but also drives higher conversion rates and loyalty.
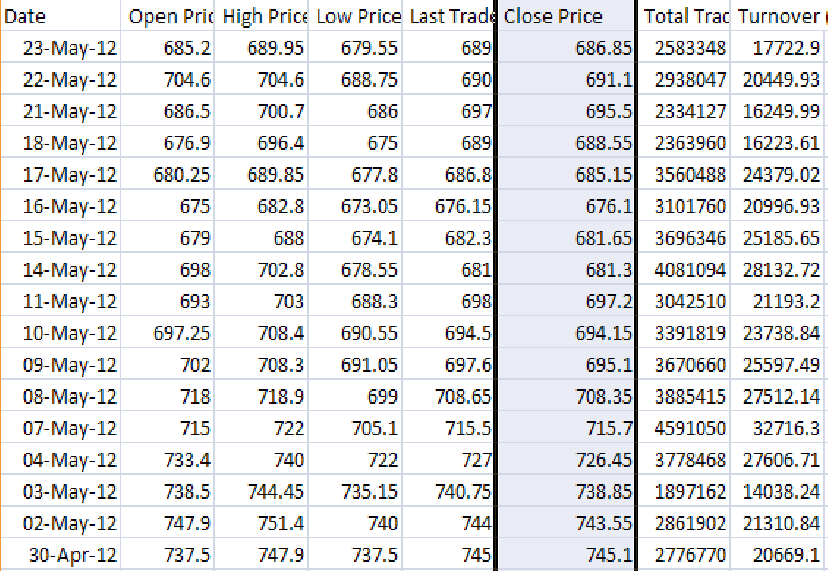
Predicting User Intent in Retail
- Using Algorithms and Data Models to Anticipate Future Behavior:
- Predictive analytics leverages historical data, machine learning algorithms, and statistical models to forecast future customer behavior. In retail, this might involve predicting what products a customer is likely to buy next, when they might make a purchase, or how they will respond to certain promotions.
Personalization Engines:
How Personalized Recommendations Based on Predicted Intent Improve Customer Experience:
Personalization engines use the insights gained from predictive analytics to deliver customized experiences to each customer. By understanding a customer’s intent—whether they’re likely to purchase, explore, or just browse—these engines can suggest products, content, or offers that are most relevant to them.
Customer Segmentation:
Grouping Users Based on Similar Behaviors or Intents for Targeted Marketing
Customer segmentation involves dividing the customer base into distinct groups based on shared characteristics, behaviors, or intents. For example, one segment might include price-sensitive shoppers, while another includes high-spenders looking for premium products.
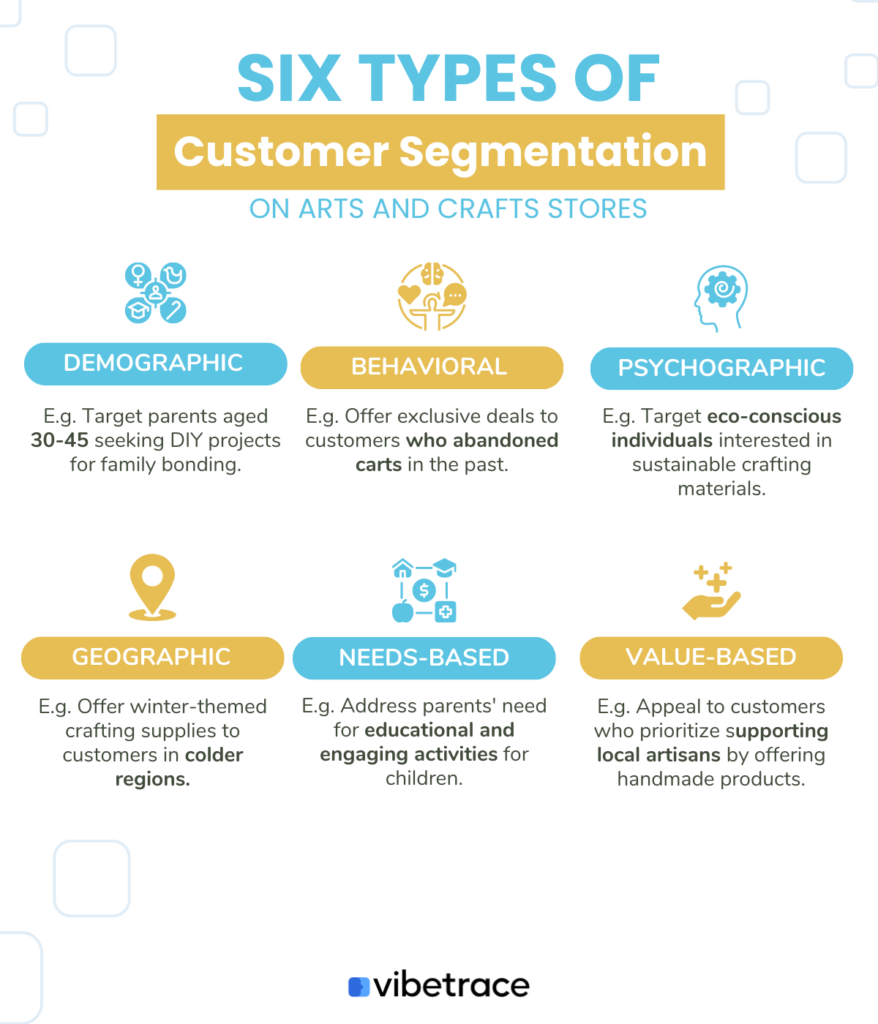
Real-Time Analytics:
Using Real-Time Data to Make On-the-Spot Predictions and Adjustments:
Real-time analytics enables retailers to analyze customer behavior as it happens, allowing for immediate predictions and responses. For example, if a customer is browsing high-ticket items, real-time analytics might trigger a special discount offer or connect them with a live sales agent.
Data Sources for Detection & Prediction
Internal Data
- User Activity Logs, Purchase History, and CRM Data: Internal data includes all the information generated from a retailer’s own systems. User activity logs capture interactions on websites or apps, such as pages visited, time spent, and clicks.
- Purchase history provides insights into what products a customer has bought, their frequency of purchases, and preferred payment methods. CRM (Customer Relationship Management) data consolidates all interactions and transactions with customers, offering a comprehensive view of customer preferences, behaviors, and past interactions.
External Data
- Social Media Signals, Market Trends, and Competitive Analysis: External data comes from outside the retailer’s direct control but is equally valuable in understanding customer behavior. Social media signals, such as likes, shares, and comments, provide insights into customer interests and sentiment.
- Market trends data helps retailers understand broader industry shifts and customer demand changes. Competitive analysis involves monitoring competitors’ offerings, pricing strategies, and customer engagement tactics.
Integrating Multiple Data Sources
- Combining Internal and External Data for More Accurate Predictions: The true power of data-driven prediction lies in integrating internal and external data sources. Combining user activity logs and purchase history with social media signals and market trends, retailers can create a more holistic and accurate picture of customer intent.
- For example, a sudden spike in social media mentions of a product category, combined with an uptick in related search activity on the retailer’s site, could indicate a surge in demand, prompting the retailer to adjust inventory or marketing strategies in real-time.
Do you like this article?
Join our CX for Retail dedicated newsletter!
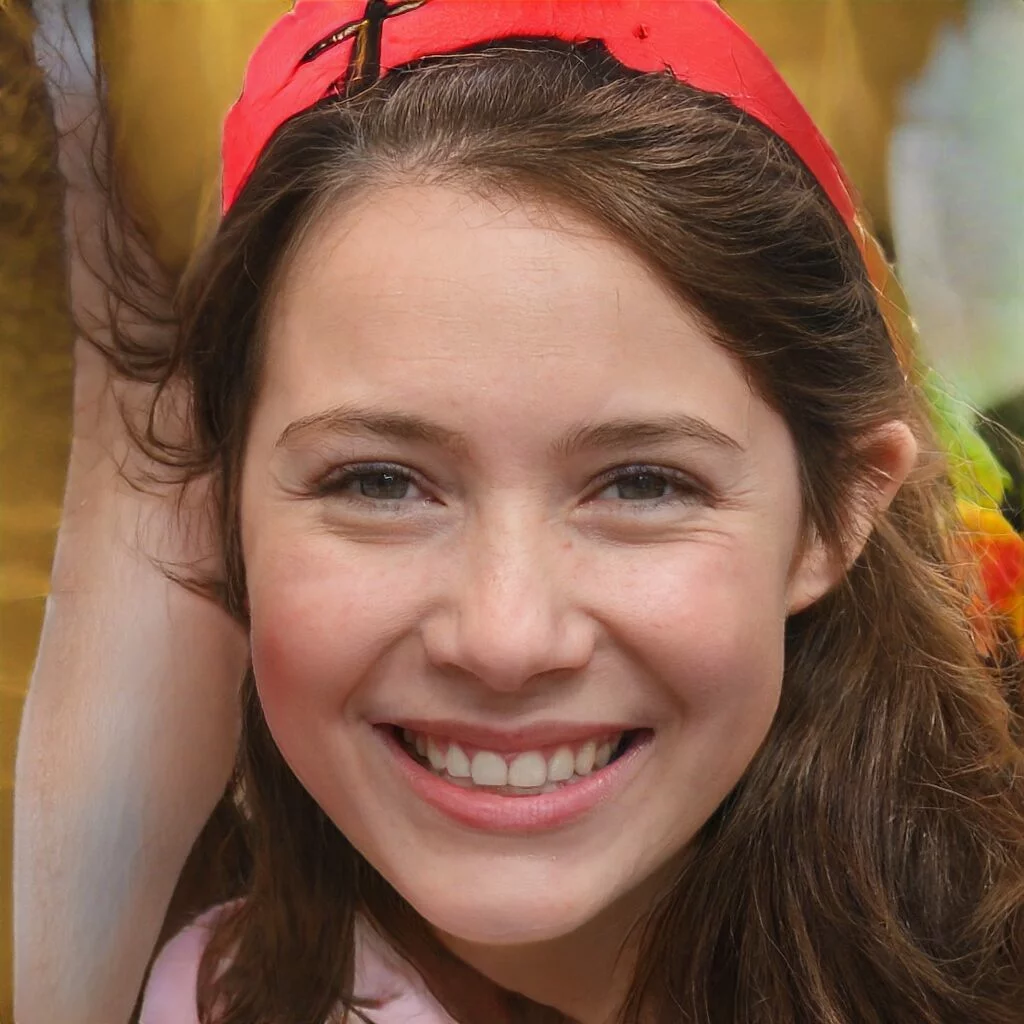
Stay connected to what’s really important to optimize your digital revenues.
By clicking the button, you accept our Terms & Conditions. Also you will need to confirm your email address.
Challenges and Considerations
Data Privacy
- Data Privacy Regulations: In the context of retail, collecting and analyzing customer data must be done in compliance with data privacy laws like GDPR (General Data Protection Regulation) in Europe and CCPA (California Consumer Privacy Act) in the United States. These regulations are designed to protect consumers by ensuring that their personal data is collected, processed, and stored securely and with their consent.
- Ethical Considerations: Retailers must strike a balance between gathering sufficient data to make accurate predictions and respecting user privacy. This involves obtaining explicit consent from customers before collecting their data, being transparent about how the data will be used, and giving customers control over their data (e.g., allowing them to opt-out of data collection). Ethical data practices not only help retailers stay compliant but also build trust with their customers, which is crucial for long-term loyalty.
Accuracy of Predictions
Handling the Limitations and Potential Inaccuracies in Predicting Intent
- Challenges in Prediction: While predictive analytics can be powerful, it’s not foolproof. Predictions are based on historical data and patterns, which might not always accurately reflect future behavior. For instance, external factors like sudden market changes or personal circumstances can cause deviations from predicted outcomes.
- Managing Inaccuracies: Retailers should acknowledge the limitations of their predictive models and continuously refine them based on new data. This might involve using a combination of different models, regularly updating algorithms, and validating predictions against real outcomes. It’s also essential to have fallback strategies in place when predictions don’t align with actual customer behavior, such as offering alternative recommendations or gathering more data to refine the prediction.
Technical Challenges
Integrating Various Data Streams and Maintaining Real-Time Processing
- Data Integration: One of the biggest technical challenges in predicting user intent is integrating data from multiple sources, both internal (like CRM systems and purchase history) and external (such as social media and market trends). These data sources often have different formats, structures, and update frequencies, making integration complex.
- Real-Time Processing: To predict user intent accurately and timely, retailers need to process and analyze data in real-time. This requires robust IT infrastructure capable of handling large volumes of data quickly and efficiently. Technical challenges include ensuring low latency, managing data flow, and maintaining system reliability.
- Scalability: As a retailer’s customer base grows, the volume of data increases, requiring scalable solutions that can accommodate more data without compromising performance. Ensuring that the system remains responsive and accurate as data scales is a significant challenge that requires ongoing investment in technology and resources.
Case Studies and Examples
Walmart’s Predictive Analytics:
- Overview: Walmart uses predictive analytics to anticipate customer needs and optimize inventory. It uses data from past purchases, weather patterns, and even local events, Walmart can predict demand for certain products and ensure they are stocked accordingly. This approach helps Walmart reduce waste, improve customer satisfaction, and maximize sales.
- Key Features:
- Integration of diverse data sources, including external data like weather forecasts.
- Real-time adjustments to inventory and pricing based on predicted demand.
- Focus on operational efficiency alongside enhancing customer experience.
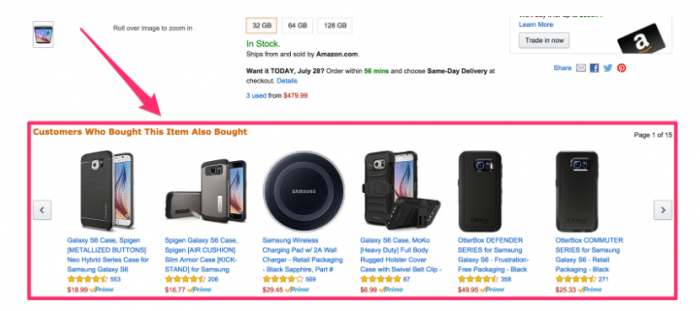
. Overreliance on Historical Data:
- Pitfall: Relying too heavily on historical data can lead to inaccurate predictions, especially in rapidly changing markets. If a retailer doesn’t account for new trends or sudden changes in consumer behavior, they might miss out on emerging opportunities.
- Avoidance Strategy: Continuously update predictive models with real-time data and incorporate external factors such as market trends, economic shifts, and social changes. This ensures that predictions remain relevant and accurate.
2. Ignoring Data Privacy Concerns:
- Pitfall: Collecting and using customer data without proper consideration of privacy can lead to legal issues and loss of customer trust. Retailers who fail to comply with data protection regulations (like GDPR or CCPA) risk penalties and damage to their reputation.
- Avoidance Strategy: Ensure all data collection practices are transparent and compliant with regulations. Provide customers with control over their data, including clear opt-in/opt-out options, and communicate how their data will be used to enhance their experience.
3. Lack of Integration Across Channels:
- Pitfall: Failing to integrate data across all customer touchpoints (online, in-store, mobile, etc.) can result in a fragmented understanding of customer intent. This leads to inconsistent experiences and missed opportunities to engage customers effectively.
- Avoidance Strategy: Invest in omnichannel integration to ensure that customer data from all channels is unified and accessible. This approach allows for a more comprehensive understanding of customer intent and enables seamless, personalized experiences across all touchpoints.
4. Inadequate Testing and Validation:
- Pitfall: Deploying predictive models without thorough testing can lead to incorrect recommendations or missed opportunities, potentially frustrating customers and harming sales.
- Avoidance Strategy: Implement a rigorous testing and validation process for all predictive models. Use A/B testing, continuous monitoring, and iterative improvements to ensure that the models deliver accurate and valuable insights.
Conclusion
To stay competitive in today’s fast-paced retail landscape, understanding user intent isn’t just a nice-to-have—it’s essential.
Retailers who can accurately detect and predict what their customers want are better positioned to provide personalized experiences, increase sales, and build long-term loyalty.
Investing in advanced technologies like AI, machine learning, and real-time analytics enables you to tap into the rich insights hidden in customer data.
These tools help you anticipate customer needs, tailor your offerings, and engage shoppers in ways that resonate with them personally.
Don’t let your competitors get ahead.
You can deliver the right message, at the right time, to the right customer—keeping your brand top of mind and driving sustained growth.
Now is the time to invest in the technologies and strategies that will ensure your retail business thrives in an increasingly competitive market. Vibetrace can help with this!